Researchers in Switzerland and Australia have used machine learning to crack the code governing charge transfer and colour emission in chains of molecules.
Exciton Science
The process of creating OLEDs can involve a significant amount of experimentation to find the right combination of materials and the right fabrication techniques that will result in efficient and stable devices. Developers may need to test various materials and processing methods, and make adjustments to optimize the performance of the OLEDs.
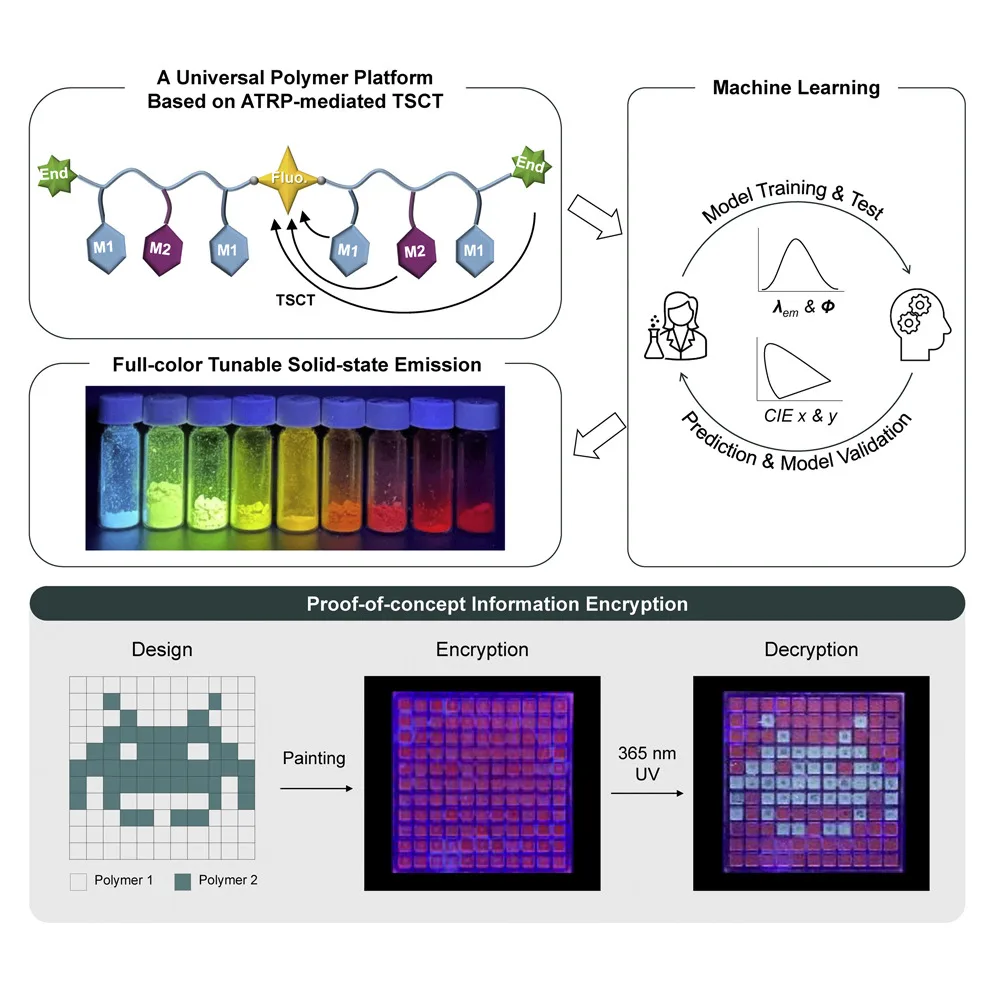
However, with the help of machine learning, the researchers can speed up this process and make it more efficient. Machine learning can be used to analyze large amounts of data and make predictions about how different materials and fabrication methods will affect the performance of OLEDs. This can help developers identify promising materials and fabrication methods more quickly, and make fewer mistakes in the process of developing OLEDs. The researchers developed a versatile and simple platform based on through-space charge transfer (TSCT) polymers that can produce full-color-tunable emission. They used a machine learning model to aid in the development of these polymers. The researchers also showed how these TSCT polymers can be used as a general design platform for solid-state stimuli-responsive materials with high-contrast photochromic emission, this means that the polymers can change color in response to different stimuli.
Reference
Suiying Ye, Nastaran Meftahi, Igor Lyskov, Tian Tian, Richard Whitfield, Sudhir Kumar, Andrew J. Christofferson, David A. Winkler, Chih-Jen Shih, Salvy Russo, Jean-Christophe Leroux, Yinyin Bao. Machine learning-assisted exploration of a versatile polymer platform with charge transfer-dependent full-color emission. Chem, 2023; DOI: 10.1016/j.chempr.2022.12.003