Researchers have developed a generative deep learning (Gen-DL) model capable of designing organic molecules with specific optical properties such as absorption/emission peak positions, photoluminescence (PL) quantum yield, and PL lifetime. The model was trained on an extensive experimental database containing 71,424 molecule/solvent pairs.
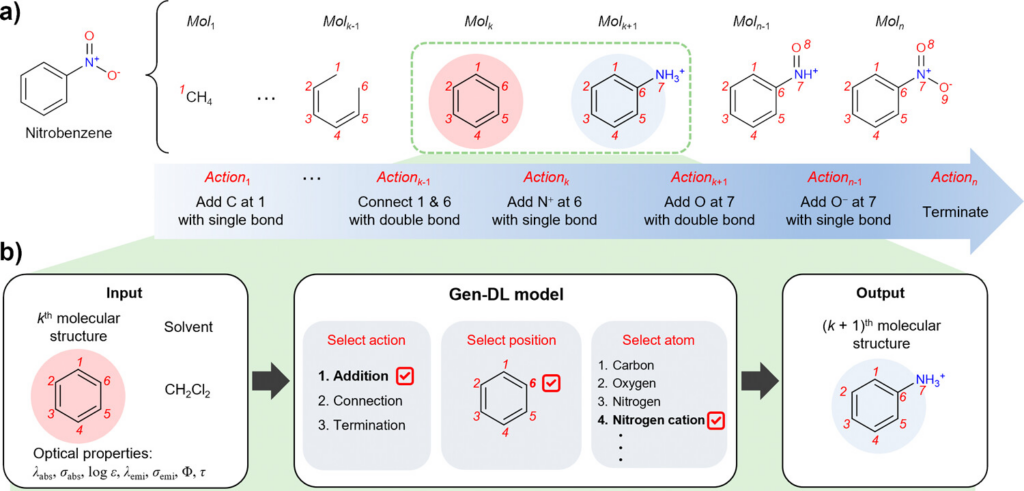
The Gen-DL model can generate molecules with properties tailored for various display technologies, such as OLEDs. For example, it can create molecules with narrow emission bandwidths, which are desirable for high-color-purity displays. It can also design molecules for bioimaging and photovoltaics, expanding its utility beyond display technologies.
Accordig to the researchers, from the Department of Chemistry and Research Institute for Natural Science, Korea University, this model accelerates the discovery process by generating molecules from scratch or by modifying existing structures, leveraging deep learning to explore a vast chemical space without relying solely on human expertise. It integrates solvent effects and other chemical principles into the design process, making it more reliable and practical for real-world applications.
The paper has specific examples where the Gen-DL model successfully designed molecules used in near-infrared imaging dyes and OLED emitters,
Reference
Han, M., Joung, J. F., Jeong, M., Choi, D. H., & Park, S. (2024). Generative Deep Learning-Based Efficient Design of Organic Molecules with Tailored Properties. ACS Central Science. https://doi.org/10.1021/acscentsci.4c00656